Maximizing Business Success with Expert Image Annotation for Machine Learning
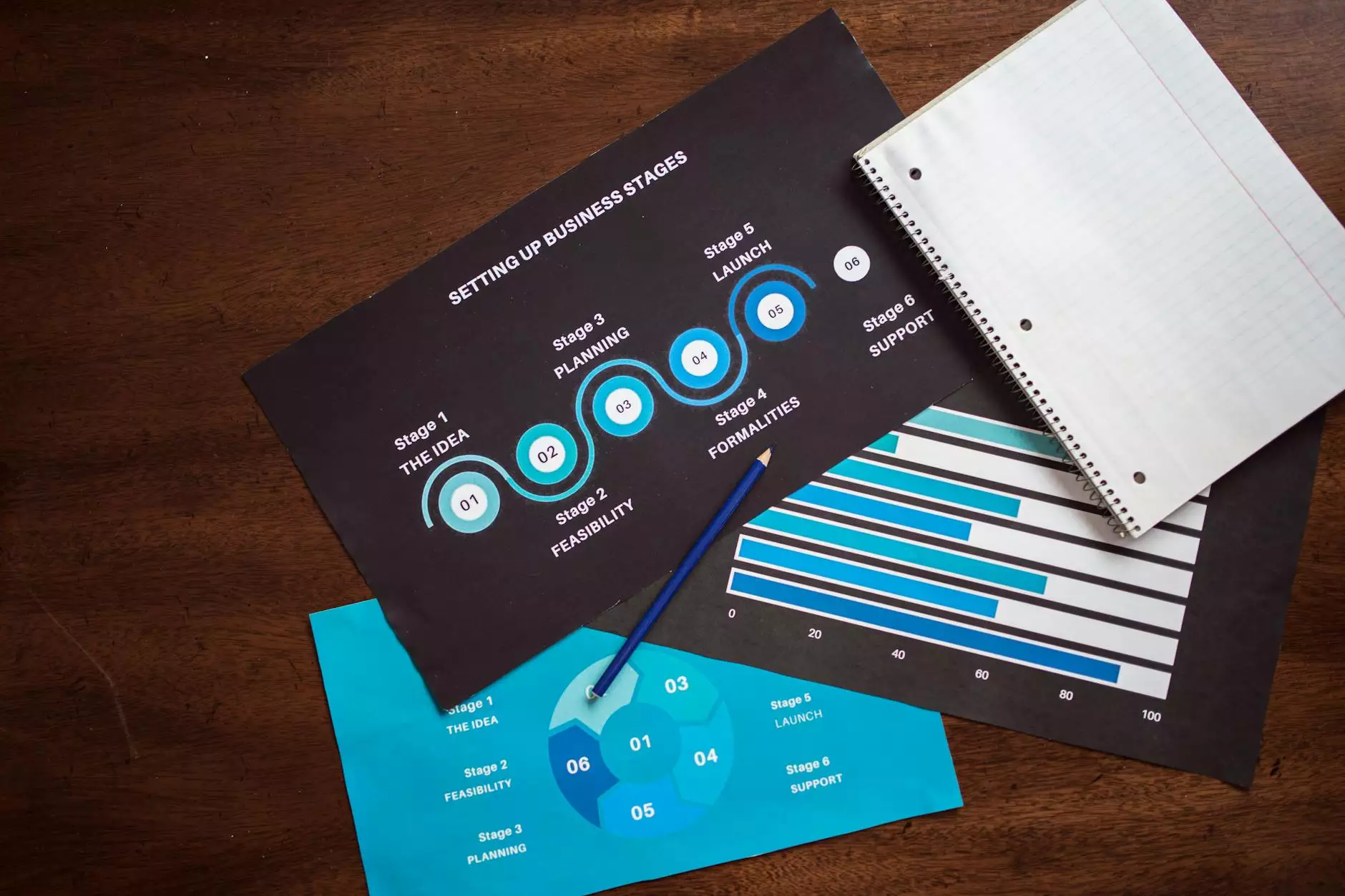
In the rapidly evolving landscape of software development, businesses are increasingly turning to machine learning (ML) to automate processes, derive insights, and innovate at a faster pace. At the heart of successful machine learning applications lies a critical, yet often overlooked component: image annotation for machine learning. This process is the backbone of creating high-quality datasets, enabling AI models to perform with higher accuracy and reliability.
Understanding the Importance of Image Annotation in Machine Learning
Image annotation involves labeling or marking features within an image—such as objects, boundaries, or specific attributes—so that machine learning algorithms can learn to identify and interpret visual data. This step is fundamental because the quality of annotations directly impacts the effectiveness of computer vision models.
Business applications leveraging image annotation are vast and varied, including autonomous vehicles, security surveillance, healthcare diagnostics, retail inventory management, agriculture technology, and more. The ability to precisely annotate images translates into superior AI performance, which in turn leads to more dependable and insightful predictions, operational efficiencies, and competitive advantages.
Why Businesses Need High-Quality Image Annotation for Machine Learning
In today’s competitive market, accuracy and efficiency are non-negotiable. Here's why investing in professional image annotation for machine learning is vital for your business:
- Enhanced Model Precision: Accurate annotation ensures models recognize objects correctly, reducing errors and false positives.
- Faster Model Development: High-quality annotations facilitate training convergence, shortening the time to deploy new AI solutions.
- Reduced Costs: Precise labeling minimizes the need for re-annotations, saving resources and time.
- Scalability: Professional annotation services enable handling large datasets efficiently, supporting extensive AI projects.
- Industry Compliance and Data Privacy: Qualified annotators adhere to strict data handling regulations, ensuring confidentiality and ethical standards.
Hence, businesses seeking to leverage AI to disrupt or innovate within their industries must prioritize exceptional image annotation practices.
The Process of Image Annotation for Machine Learning: A Step-by-Step Guide
Understanding the workflow behind image annotation helps clarify its significance and complexity. Here are the essential phases involved:
1. Data Collection and Preparation
Gathering raw images relevant to the specific use case, ensuring diversity and quality to train robust models.
2. Annotation Strategy Development
Defining annotation categories, including labels, bounding boxes, polygons, or semantic segmentation depending on model requirements.
3. Annotation Execution
Using specialized tools or outsourcing to trained annotators to precisely label images. This step demands consistency, attention to detail, and domain expertise.
4. Quality Assurance and Validation
Implementing review processes to verify annotation accuracy, rectify errors, and ensure dataset integrity.
5. Dataset Finalization and Integration
Consolidating labeled data into machine-readable formats, ready for training models.
6. Model Training and Optimization
Using annotated datasets to train ML algorithms, followed by tuning for improved performance.
Key Technologies and Tools in Image Annotation for Machine Learning
Effective image annotation relies on a combination of cutting-edge tools, annotation standards, and automation techniques:
- Labeling Platforms: Software like Labelbox, CVAT, RectLabel, and VGG Image Annotator enable efficient annotation workflows.
- Automation and AI-assisted Annotation: Employing AI tools to pre-label images, which human annotators then verify and refine, accelerating the process.
- Annotation Standards: Consistent use of formats such as COCO, Pascal VOC, YOLO, and XML ensures compatibility across ML frameworks.
- Quality Control Mechanisms: Multi-stage review systems and collaborative workflows to maintain annotation quality.
Choosing the Right Partner for Image Annotation Services
Partnering with a reputable, experienced service provider like Keymakr can be transformative for your AI initiatives. Consider these factors when selecting an annotation partner:
- Expertise in Your Industry: Domain knowledge ensures accurate labeling aligned with industry-specific requirements.
- Quality Assurance Processes: Robust validation and review workflows are essential for high-quality datasets.
- Scalability and Turnaround Time: The ability to handle large projects efficiently with fast delivery capabilities.
- Data Security and Confidentiality: Compliance with GDPR, HIPAA, and other protections safeguards sensitive information.
- Technological Capabilities: Investment in cutting-edge annotation tools and AI-assisted workflows enhances productivity.
Partnering with experts like Keymakr ensures your datasets are meticulously prepared, augmenting your business’s AI impact significantly.
Business Benefits Achieved Through Professional Image Annotation for Machine Learning
Embracing professional annotation services yields tangible benefits:
- Higher Accuracy Models: Better annotations translate into models that perform reliably in real-world scenarios.
- Operational Efficiency: Reduces time-to-market for new AI-driven products.
- Cost Savings: Less retraining and re-labeling reduces overall project expenses.
- Competitive Edge: Superior data quality empowers businesses to lead in their markets with innovative AI solutions.
- Enhanced Customer Satisfaction: Accurate AI outputs improve user experiences and trust.
- Risk Mitigation: Precise data reduces the risk of model bias and errors, safeguarding your reputation.
Future of Image Annotation in Business and AI Innovation
The future of image annotation for machine learning is promising, driven by technological advancements such as:
- AI-Driven Annotation Tools: Increasing automation will reduce manual efforts and improve consistency.
- Increased Use of 3D and Video Annotations: Expanding capabilities to encompass complex data types.
- Integration with Augmented Reality (AR) and Virtual Reality (VR): Enabling immersive data annotation experiences.
- Enhanced Annotation Standards and Protocols: Promoting interoperability and data sharing across industries.
- Real-Time Data Labeling: Supporting live systems like autonomous vehicles and surveillance.
By embracing these innovations, businesses can unlock unprecedented AI capabilities, transform their operational models, and stay ahead in competitive markets.
Conclusion: Why Your Business Can’t Afford to Overlook Image Annotation for Machine Learning
In the complex realm of software development and AI, image annotation for machine learning is not just a preparatory step—it is the foundation upon which successful AI models are built. Investing in professional annotation services, such as those offered by Keymakr, equips your enterprise with high-quality datasets crucial for achieving accuracy, efficiency, and innovation.
Whether you are developing autonomous systems, healthcare diagnostics, retail automation, or any other AI-powered solution, superior data annotation determines your success trajectory. As the landscape of AI continues to evolve rapidly, staying ahead means committing to best practices in data preparation. The future belongs to those who understand that quality annotation is a strategic business asset—one that empowers smarter decisions, better products, and stronger market positioning.